Unraveling the Synergy between Machine Learning and 2D Materials
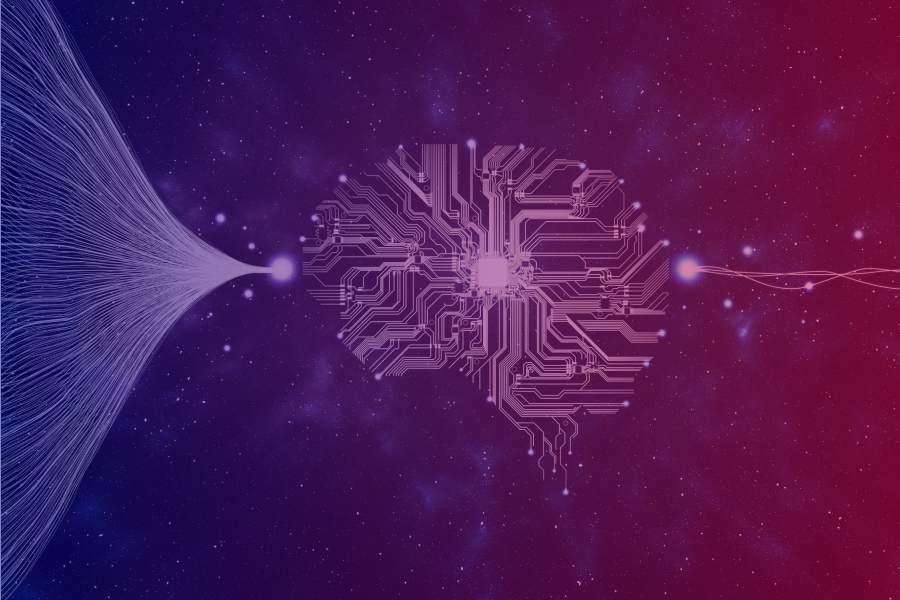
Article based on the content of the paper:
B. Lu, Y. Xia, Y. Ren, M. Xie, L. Zhou, G. Vinai, S. A. Morton, A. T. S. Wee, W. G. van der Wiel, W. Zhang, P. K. J. Wong. “When Machine Learning Meets 2D Materials: A Review” Adv. Sci. 2024, 2305277. https://doi.org/10.1002/advs.202305277
The integration of Machine Learning (ML) with the study of 2D materials represents a frontier in material science, combining advanced computational models with intricate material properties. This synergy not only accelerates the discovery of novel materials but also enhances the understanding of their complex behaviours.
The application of Machine Learning in 2D material research is pivotal for the AI technology sector. By employing algorithms capable of predicting material properties and behaviours, researchers can drastically reduce the time and cost associated with experimental and computational studies. This approach not only speeds up the innovation cycle in materials science but also offers a blueprint for leveraging AI in other domains of scientific inquiry, making it a cornerstone for technological advancements and a catalyst for industrial innovation.
The study outlined significant advances in using Machine Learning to predict and analyze the properties of 2D materials. Machine learning models have been demonstrated to effectively predict material characteristics such as bandgaps, thermal properties, and mechanical stability, thereby aiding in the design of new materials with desired functionalities. These capabilities are expected to evolve, with Machine Learning models becoming increasingly sophisticated, allowing for more accurate predictions and efficient material design processes. Moreover, the research highlights the potential of Machine Learning to manage large datasets, which is crucial in identifying trends and making predictions in the vast, complex parameter spaces of material properties.
This research aligns with the strategic goals of HYBRAIN, particularly in merging theoretical and experimental methodologies in innovative ways.
With contributions from Wilfred van der Wiel, HYBRAIN coordinator and backed by funding from HYBRAIN, the study sets a precedent for employing artificial intelligence to enhance material science research.
The insights gained are paving the way for interdisciplinary projects that leverage AI, potentially leading to transformative advancements across various industries, including electronics and renewable energy.