Abu Sebastian (IBM, HYBRAIN Innovation Manager team) elected IEEE Fellow for his contributions to the In-Memory Computing field
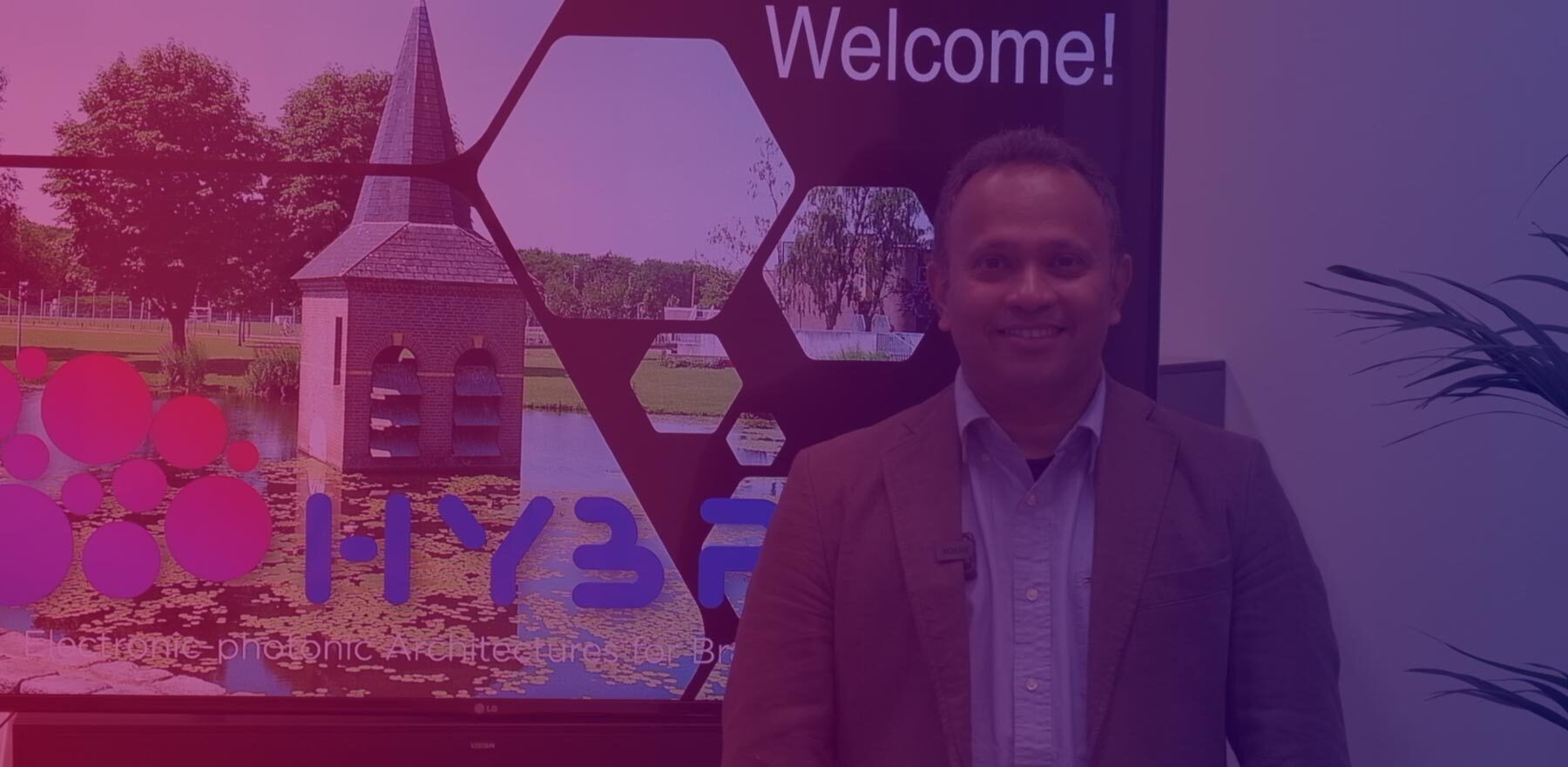
Thanks to his contributions to the field of in-memory computing, Abu Sebastian (IBM researcher and HYBRAIN Innovation Manager team) was recently elected Institute of Electrical and Electronics Engineers (IEEE) fellow.
A designation known as an IEEE Fellow is only awarded to a small group of members who have made remarkable contributions to one or more of the IEEE’s fields of interest.
Moore’s law decline
The exponential growth in computing power, speed and energy efficiency of the last decade has been correctly predicted by Moore’s law: roughly every two years, the number of transistors on microchips will double.
A silicon atom is 0.2 nanometers wide, while the transistor gate, the part of the transistor through which electrons flow as electric current, is now approaching a width of just 2 nanometers. This means that transistors are approaching the point where they are simply as small as we can ever make them and have them still function. The way we have been building and improving silicon chips and the Moore’s law itself are coming to their final iteration.
This has highlighted the speed and energy consumed during the transfer of data between the memory and the CPU. In fact, in data-intensive computation, such as deep learning, data communication between these two is the main cause of latency and energy consumption.
As the size and complexity of AI models increase, so do their energy efficiency and speed requirements.
But with Moore’s law decline, a new field has emerged to try to increase AI models’ speed and energy efficiency: In-memory computing (IMC).
In-Memory Computing
With In-Memory Computing, the operations are carried out within the memory itself, as opposed to having separate compartments for memory and processing. As a result, access to stored data is sped up and there is no longer a need to move data around continually.
Building a crossbar array of “wires” with each crossing point representing a storage unit for memory is one technique to implement IMC.
Phase-change materials are a common material choice for the wires, as they can vary their electrical resistance by simply heating or cooling them.
In-memory computing and HYBRAIN
Over the past few years, there has been an ever-growing demand for innovative artificial intelligence applications dealing with the rise of big data and the Internet of Things (IoT), resulting in very large computing power and memory requirements.
Processing such huge amounts of data requires unprecedented increases in processing power, memory, communication bandwidth, and energy efficiency, which cannot be met by modern digital electronic technologies that are rapidly approaching their physical limits.
HYBRAIN aims to close this gap by providing a hybrid electronic-photonic architecture capable of providing processing power, energy efficiency, ultra-low latency and bandwidth of the Edge-AI hardware beyond the current capacities of electronic computing systems.
To do this, HYBRAIN combines the added value of the following three recent scientific developments to obtain an unprecedented technology, where the whole is greater than the sum of the parts:
- Massively parallel Photonic Convolution Processing (PCP) developed by Oxford and Münster.
- Large-scale, Analog In-Memory Computing (AIMC) based on phase-change memristive crossbar arrays developed by IBM Europe.
- High-dimensional nonlinear classification in Dopant Network Processing Units (DNPU) developed by Twente.
Matrix multiplication is the most widely used and indispensable information processing operation in artificial intelligence (AI). It is widely used in artificial neural networks (ANNs), which have been universally applied in signal processing, imaging recognition, voice recognition, real-time video analysis, and autonomous driving.
A key advantage of AIMC is the ability to perform matrix-vector multipliers (MVMs) operations in less than 100 ns.
Therefore, by seamlessly interfacing the AIMC with the PCP and DNPU, HYBRAIN will enable a whole new spectrum of edge AI applications, such as autonomous cars, smart cities, Industry 4.0 and home automation.
Scroll down to subscribe to our newsletter to stay up to date with our research activities and results.